Using Predictive Analytics to Produce Better Claims Outcomes
You have predictive analytics. Now what? Data collection is step one, but it is what you do with that data once you have it that makes all the difference. Here are some ways to assess your data quality and use your data effectively.
June 4, 2021
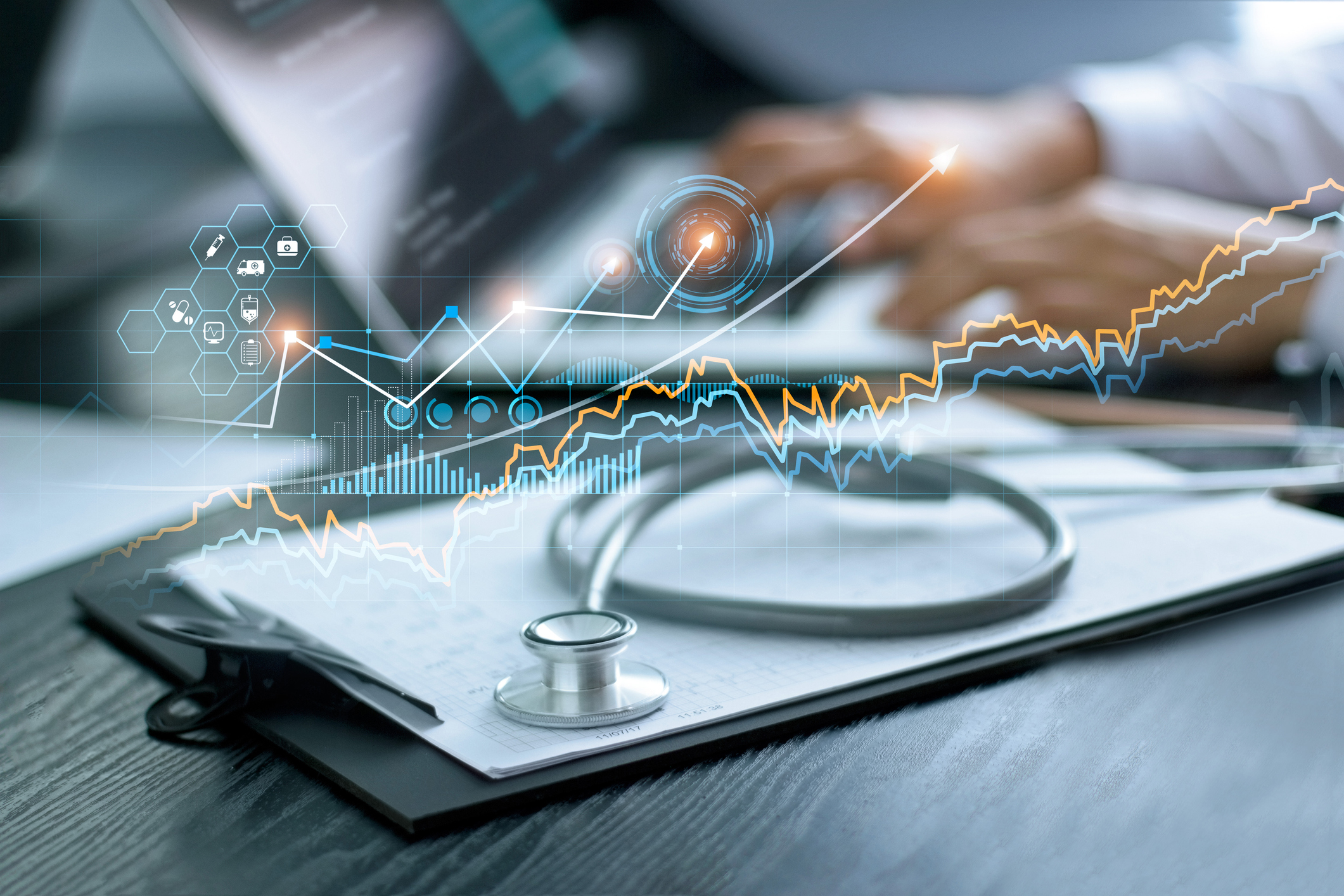
Predictive analytics are derived by extracting information from existing data sets to determine patterns and predict future outcomes and trends. It combines forecasting, classification, text analysis, machine learning and regression patterns to give you an idea of how a claim may be affected in the future. While predictive analytics do not necessarily tell you what will happen in the future, this data can certainly help us make more informed decisions.
The purpose of applying predictive analytics to claims is to identify patterns as early as possible so the right resources are deployed at the right time to achieve better than predicted outcomes. “With the prevalent availability of data, most know the role of predictive analytics, what they are and how to implement them,” said Stephen Peacock, Assistant Vice President of Claims. “What is often misunderstood is how to retrieve the most current analytics and how to use them properly once you have access to them.”
Utilizing Your Data Effectively After all of the data is retrieved, the first step is determining what data will provide the most insight into an injured worker’s claim. Specific details regarding medical history and psychological perception of the injured worker can help make the most informed decisions. For example, understanding the injured worker’s pain perception and coping skills, the expectation of a return to work, current diagnosis and treatment, while also factoring in basic details like years of employment, age, sex and co-morbidities, can aid in more accurately underwriting and pricing future risks and improving solvency in cases. These factors can also allow insurers to appropriately incentivize risk reduction, enrich the customer experience by quickly resolving service issues, and improve marketing effectiveness by tailoring products to individual preferences.
Assessing and Improving Your Data A critical review of your data measurements will govern which areas of your claims processes need adjusting. Tracking the progress of like claims can uncover blind spots, making it easier to identify why one method works more effectively than another. Using this data can refine processes, like how to utilize adjusters and supervisors most effectively. For example, placing experienced adjusters on long-term cases may lead to a better overall outcome. A further in-depth data review allows advanced tracking measures on items like how triage nurses are performing, which physicians are more proactive in their responsiveness, and which nurse case managers provide the most value. This defined data review grants proactive approaches and truly allows claims professionals to see what is working and what is not.
Considering the Benefits Several factors need to be employed to achieve the most successful outcomes for claims. Peer-to-doctor engagement, drug utilization reviews, employer commitment, constant communication with the injured worker and a stronger push for a return to work or modified duty all factor into the outcome of a claim. A commitment to utilizing predictive analytics and taking steps to improve processes can result in overall lower claim costs, better opioid control, lower expenses, faster claim closures, improved applicant satisfaction and improved reserve accuracy. All of these benefits can lead to less uncertainty resulting in a reduced possibility of moving to litigation and greater availability of insurance and assumption of risk.