Data’s Use in Identifying and Impacting High-Risk Claims
It’s an age-old issue; how do you keep your finger on the sleeper claims with creeping costs associated with medical treatments and litigation expenses? Changes in data modeling and structuring could help to more efficiently evaluate the most hazardous claims.
September 11, 2023
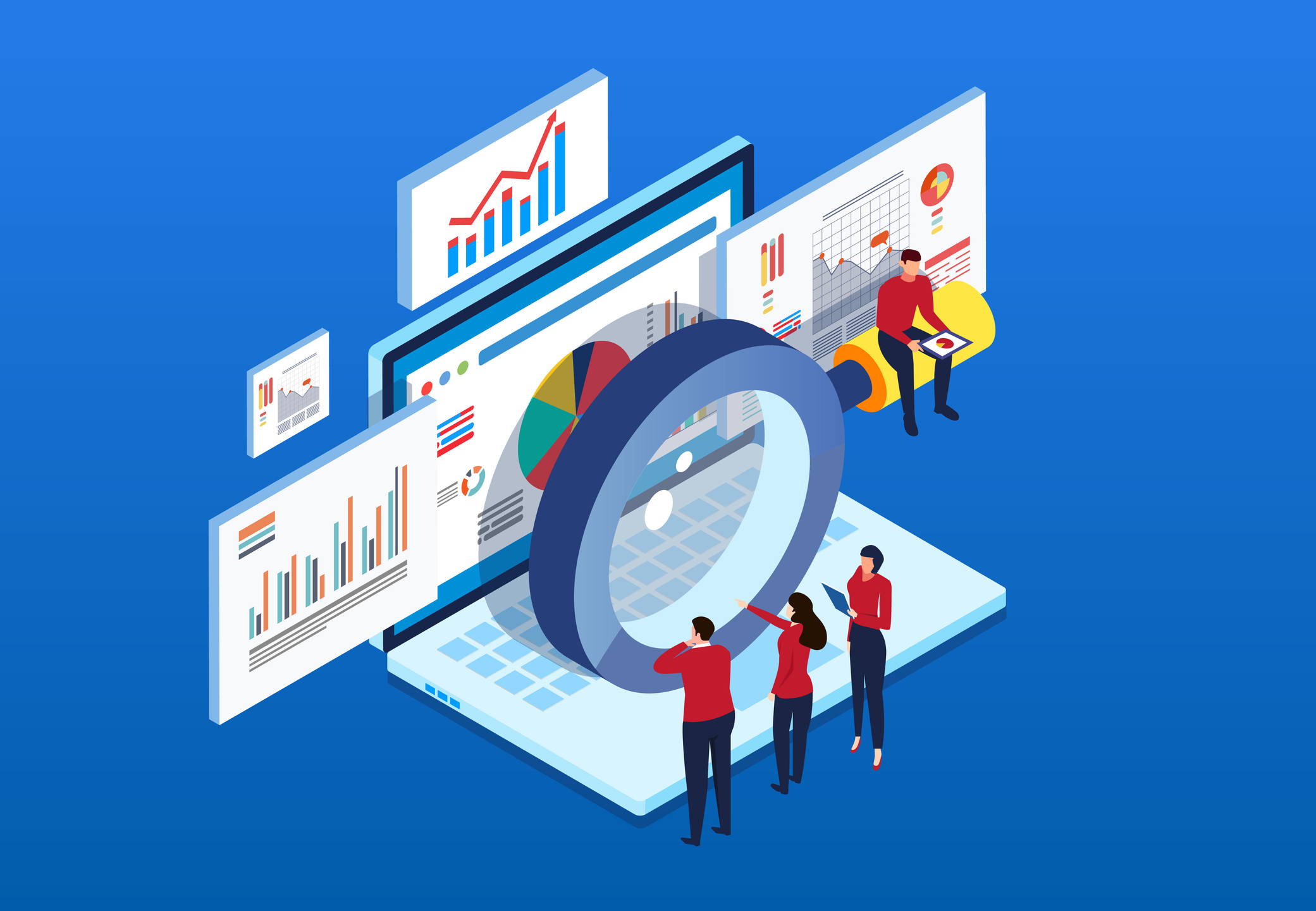
Predictive analytics may not give us a crystal ball to view the unknown, but using key data points can help to quickly identify claims that have a probability of developing into more costly claims. This can allow claims professionals to make informed decisions early in the claim, leading to better outcomes for injured workers and reducing overall claim costs. Tracking the progress of like claims with the same characteristics can help identify trends in comorbidities, litigation risks, and even industry-specific issues.
“Where insurers used to use a diary system to monitor for 90 days, they are now using predictive modeling to establish whether or not a claim is high risk based on a number of factors,” said Colleen Slyngstad, Assistant Vice President – Data Analytics at Safety National. “AI has provided an incredible opportunity by pointing us to those claims that might normally go unnoticed, allowing for early intervention and proactive decision making, and it will only continue to advance our early identification efforts.”
What are the driving factors in high-risk claims?
Claims with creeping costs, or “sleeper claims,” can develop into expensive claims as time passes because they can go unnoticed with costs that are not exponential in terms of their degree of severity. However, costs begin escalating as increased frequency and complexity of medical treatments are requested, if litigation ensues, or attempts of the injured employee to return to the workforce fail. Additionally, with more remote employees in the workforce, it can be increasingly difficult to define when an injury is work-related. With no witnesses at home office spaces, the benefit of the doubt could fall to the injured worker, allowing for the collection of workers’ compensation benefits. Third-party administrators (TPAs) will perform full investigations, but it may lead to much uncertainty around whether or not these types of accidents can arise out of employment.
On the liability side, social inflation, associated with public mistrust in large corporations, continues to drive massive jury awards. Since workers’ compensation is statutory, ultimate costs are based on regulated benefit amounts, impairment ratings, and controlled medical costs set through state fee schedules, leaving much of the industry’s volatile settlements in liability claims. Using the data collected early in the liability investigation, as well as tracking litigation related information can assist in mitigating, or possibly preventing, the high settlements that are becoming all too familiar.
How is data changing the approach to these claims?
Insurers have used data in some regard for decades, but now it is being cultivated to create predictive models that can more quickly identify potentially high-cost claims. As carriers have become adopters of claims models on severity, there is significant emphasis placed on the need to include more accurate and timely claims coding, improve text mining, build systems around structured data, and keep track of data points that identify when a claim may escalate. Data points that might be represented include:
- Anonymized injured worker’s age and type of injury
- The jurisdiction where the injury occurred
- Any potential venues for litigation
- Reputation of the employer’s return-to-work efforts
- Skill of either the defense attorney, opposing counsel, or both
However, human touch must still be a part of this process. Insurers are responsible for optimizing the data they receive to present to the claims professional to make ongoing strategic judgment calls. This data can help predict what prescribed drugs might determine the possibility of addiction, comorbidities that could impact recovery, and industry-specific data with similar trends for employees (i.e. if a group of fleet drivers carries similar risks like hypertension or obesity). For liability claims, it may predict the potential for an adverse verdict of significant costs.
What does the future look like for claims trends and data’s impact?
While major trends like social inflation have no end in sight, advances in predictive modeling can help provide a more strategic plan to address and mitigate costs associated with these liability claims. Limitations in large jury awards would prove even more beneficial, but not much headway has been made in changing the regulatory landscape. Large volumes of claims are necessary to further customize predictive modeling, so there is an incredible opportunity for growth, especially with advances in AI. The optimization that could assist in identifying and intervening quickly will only improve.